How many more coffees can our machine do before requiring maintenance? Well, that’s a fundamental question for most of the ELEDIA members. The obvious answer to this may be “we’ll know when it’s broken”. But if the machinery has a fundamental importance in the production line, will an unexpected downtime for maintenance be acceptable?
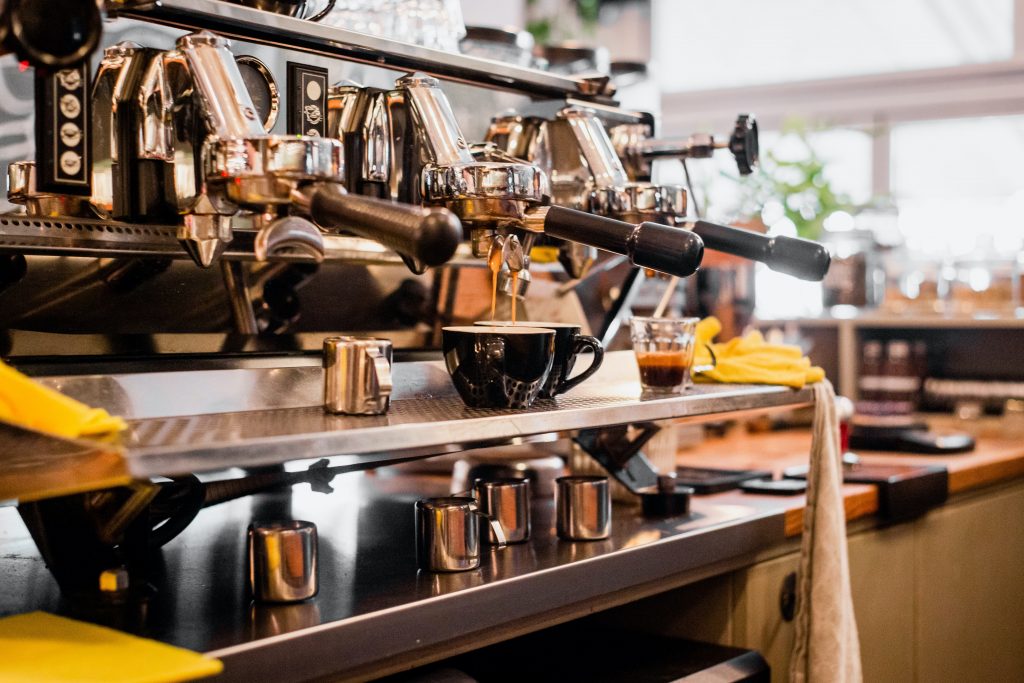
Within the Maintenance, Repair and Overhaul (MRO) terminology, the previously outlined approach (substitution/maintenance of a machinery after the failure has taken place) would be referred to as a corrective maintenance, and it is known to be a suitable strategy only if the production process can be interrupted at any time with minimum consequences (btw, that’s NOT the case for the ELEDIA coffee machine). A completely opposite strategy is to schedule the maintenance after a pre-defined number of cycles / operation hours have been carried out, following the concept of preventive or planned maintenance. As the inspections are performed on a periodical basis, this strategy has the obvious advantage of guaranteeing fixed costs. But are such costs minimum? In the era of Smart Factories and Industry 4.0, the answer to such a question may be less obvious.
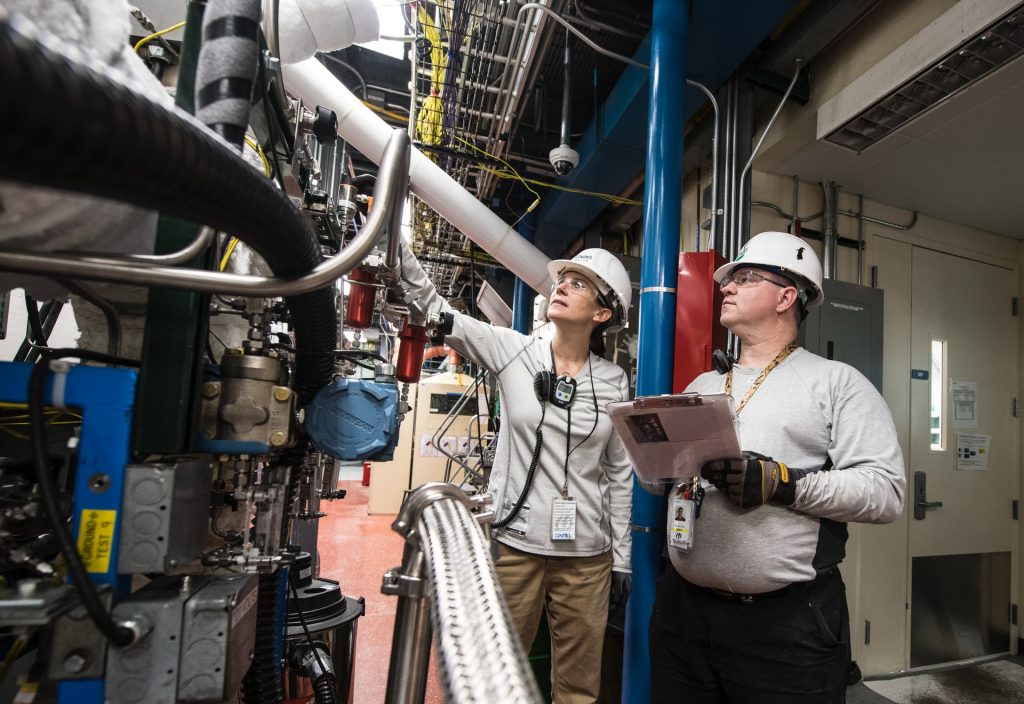
Minimizing the maintenance costs by knowing in advance when exactly a machinery or process will fail has always been the dream of industrial process managers. Predictive Maintenance actually emerged as one of the most concrete applications of Industry 4.0 to fulfill this dream. The fundamental objective of predictive maintenance is to determine the condition of a machinery / process so to define when to the maintenance is actually needed. In this sense, it differs from preventive or corrective maintenance since it is based on the actual prediction of the equipment status (data-driven prognostics) rather than on its expected average lifespan (statistic-driven prognostic) or on the detection of an already happened issue (diagnostics). The only drawback is that predicting the future does not generally look a simple task.
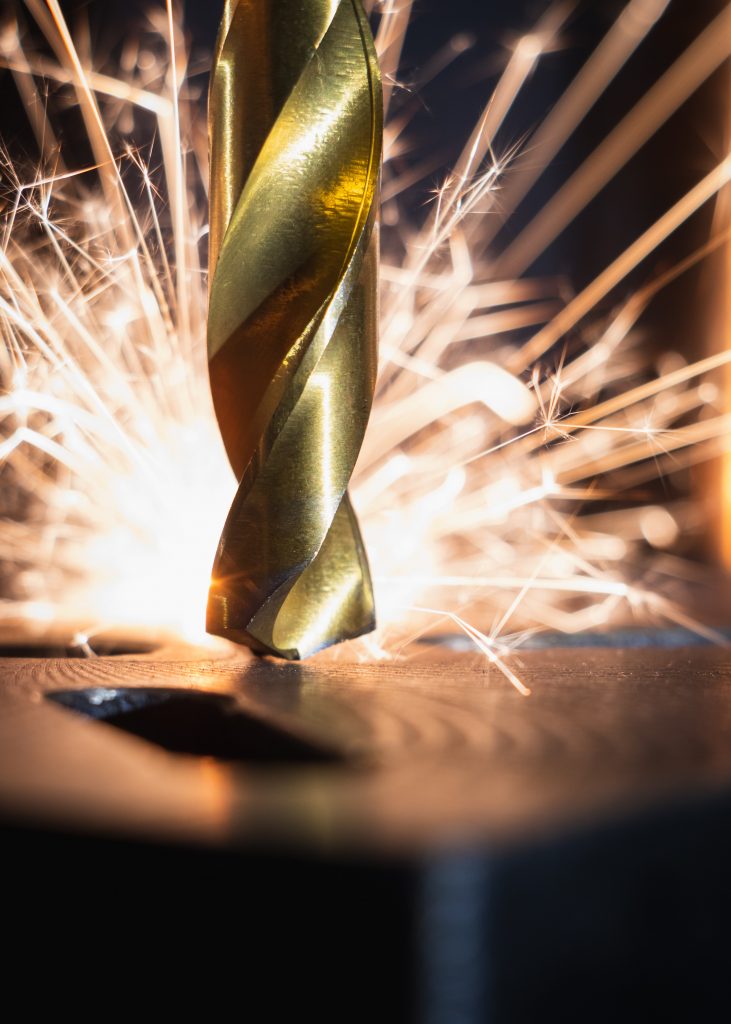
Modern automation systems are equipped with a wide variety of sensors enabling the observation of the status of machines and processes. Moreover, the availability of low-cost deployable wireless sensors and the IoT paradigm are further expanding the capability of acquiring detailed data from complex systems even operating in large factories, as well as to collect such data for further remote processing. Still, a simple question arise: can I understand how many cycles the cutting machine will perform accurately from the sound of its rotating blades?That’s where AI comes into play.
In the recent years, ELEDIA members have developed, applied, and deployed a wide set of AI methodologies for Industry 4.0 scenarios both at small- and large-scales, developing prognostics and predictive maintenance tools that have been applied both to single machines or fabrication processes and to large scale factories displaced in separated facilities. The fundamental approach followed by ELEDIA to achieve high-accuracy and efficiency predictive capabilities has been a combination of both physical insights on the actual process / machine under observation and advanced analysis and learning capabilities enabled by AI. By integrating the knowledge of the expected physical features of the investigated process (e.g., relation between pitch frequency/spectrum of the sound emitted by a rotating blade vs. rotation regime, cut material, and cut speed) and by customizing the E-AIR artificial intelligence methodologies, the possibility to achieve early detection of anomalies in industrial processes and reliable early maintenance warnings has been demonstrated. To this end, the “fusion” of Key Performance Indicators (KPI) data and information collected from several different nondestructive evaluation and nondestructive testing technologies (such as sound level measurements, acoustic analysis, pressure and vibration analysis, temperature/humidity measurements) has been a fundamental challenge to be addressed.
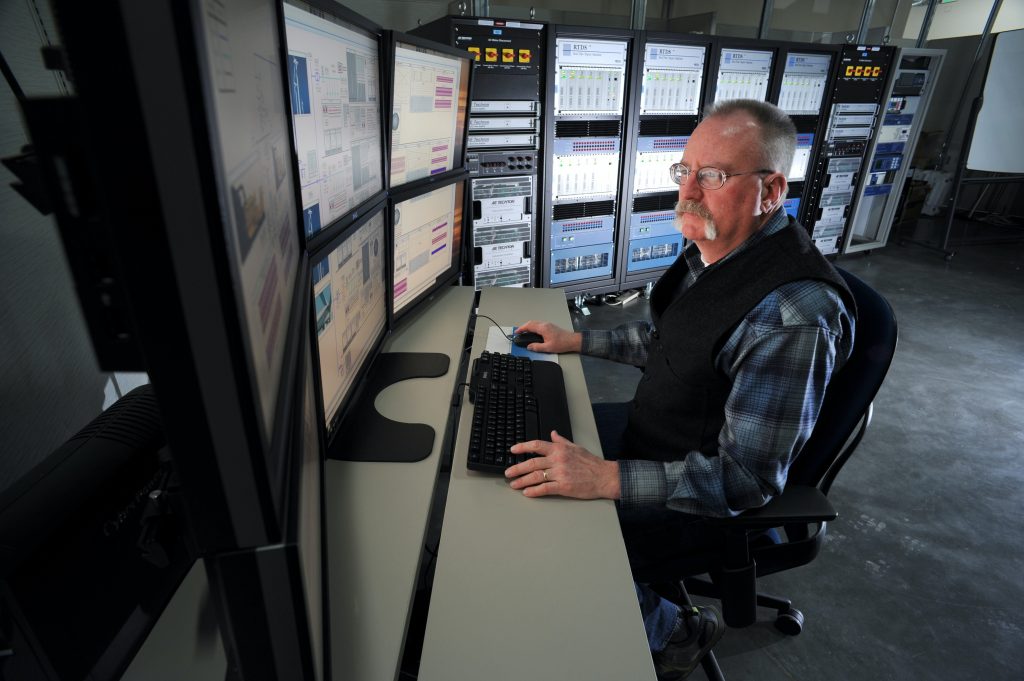
In this scenario, a recent application of the E-AIR Industry 4.0 suite has been in the field of both automatic machine health status analysis and fabrication process control in the industrial collaboration with IMA Group, a world leader in the design and manufacture of automatic machines for the processing and packaging of pharmaceuticals, cosmetics, food, tea and coffee.
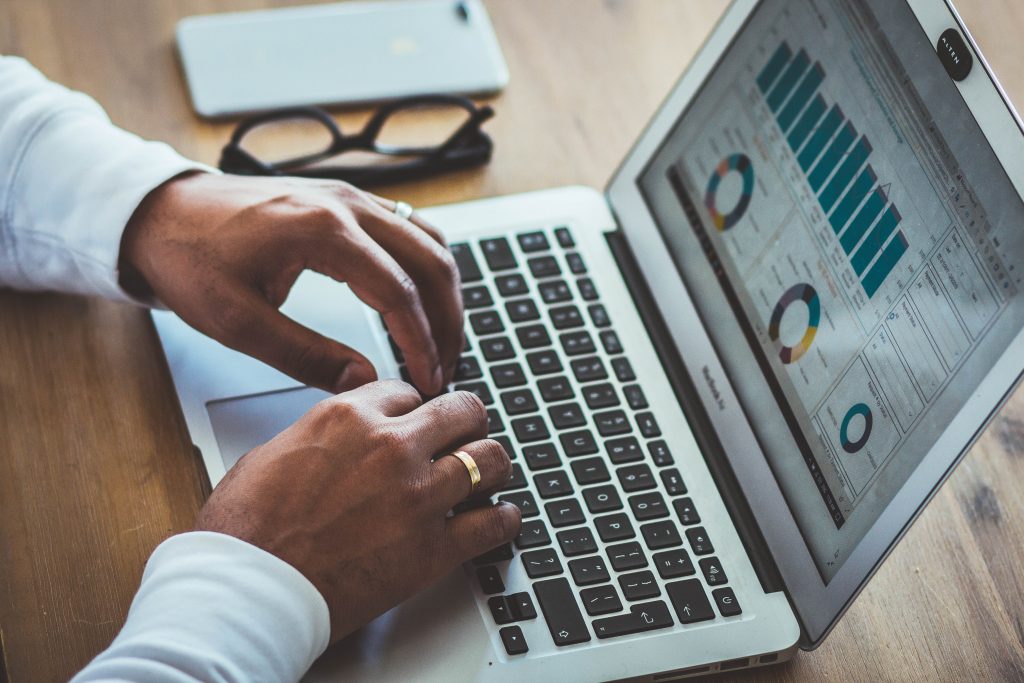
The current research efforts in ELEDIA are aimed at a further customization of the E-AIR Industry 4.0 Suite methodologies for data fusion, data analysis, and machine learning to more complex Model-Based Predictive Maintenance scenarios. In this framework, the possibility to self-learn the Digital Twin counterpart of an actual machinery system / process has already been demonstrated by ELEDIA members in practical industrial scenarios, and its generalization to handle large-scale multi-factory problems is currently under development.
So, next time someone will ask “How many more coffees can our machine do before requiring maintenance”, E-AIR will be there to help.
More Info
For additional information on our Industry 4.0 AI demos and software suites, please contact us at contact@eledia.org.
Related Projects
- EVOKE Project http://www.eledia.org/showcase/evoke/
- EYESHADE Project http://www.eledia.org/showcase/eyeshade/
- FAKE Project http://www.eledia.org/showcase/fake/